Article
Real world effective data management
Everyone seems to agree that data is a critical resource for serious financial institutions. Is it not curious then that in so many organisations data is not treated with the importance it deserves: quite often data is processed manually, is late, gets lost, is incomplete, or inconsistent or incorrect. These are particularly urgent problems when it comes to external data required for processes from portfolio management, compliance and risk management to accounting and reporting.
Regulatory pressure and client demand have greatly increased the need for more transparency into investment manager activities, which in turn has increased demand for more and increasingly detailed information on investments. As the picture below demonstrates this can give rise to quite some complexity. Especially as the different roles that each of the market participants can play in any given situation can change.
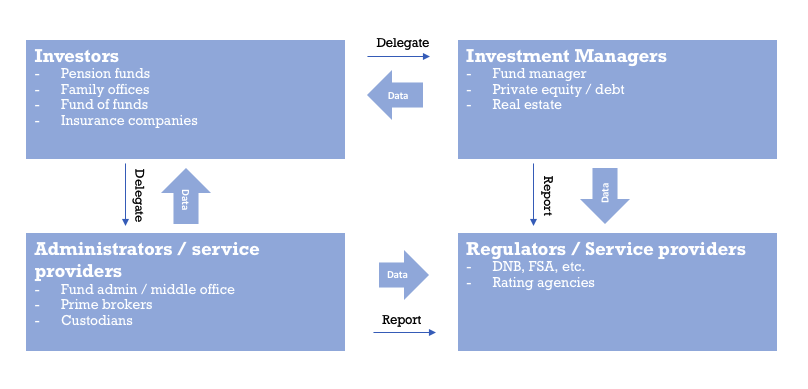
Obviously this is an oversimplified view of the market. But organisations do exchange massive amounts of data amongst each other sometimes in different capacities. A lot of the data that is changing hands is quite similar in nature, in the end everyone is interested in the same things: market data, risk/performance, portfolio holdings (in the broadest sense) and transactions. So we must have solved this, right?
Not quite: some standards exist and systems have been implemented to automate stable data exchange, but Email, Excel are still prevalent as a primary means of data exchange. This wreaks operational havoc and creates security nightmares. Let’s explore these themes a little bit further.
Standards, maybe not so standard after all
Apart from well known standards like SWIFT and FIX, which cater to data exchange of highly stable and simple data, very little standardisation has been achieved on wider industry level.
There are many initiatives that aim to achieve this, for example: Solvency 2 TPT, Inrev, ILPA, FundsXML, etc. While these have found adoption in some products and services, they generally do not play a large role in manual data exchange processes. What makes these standards cumbersome in use is the technical handling; formats are often complex and unwieldy (think XML or very large Excel sheets). Also, these standards usually prescribe a layout in terms of columns and content but organisations generally have no way to actually enforce or validate any of the more in-depth data structures (like classifications, code schemes, formats, etc.).
In a lot of cases capabilities to effectively leverage standards are missing or standards are ‘abused’ and thus lose their power to truly harmonise data exchange.
Systems? Not so fast
Many data management system vendors will promise to solve all your data management problems. Ranging from technical solutions to super smart AI, machine learning and robotics applications, systems like these are expensive and require large investments in license fees and implementation up front with little to no guarantee that they will actually make practical operational problems go away.
Data management systems are good at processing large amounts of structured and stable data: e.g. end-of-day market data feeds, accounting snapshots or transactions. They are less well suited to exception handling at every step in the process, dealing with volatile layouts, enabling manual intervention or providing a user friendly window into the data and the process.
Furthermore, traditional delivery models of these system require long implementation projects before actual business value is generated. Changing the system afterwards or getting new functionality rolled out is difficult.
Data management systems can play an effective role in automating simple, stable and structured data feeds. But they are ill-equipped to enable flexibility and agility on the end-user side.
Excel, email and PDF, things of the future?
A short time-to-market, providing a good service to clients and to meet regulatory deadlines requires rapid onboarding of new or changed data sources to be integrated into operational and reporting systems. Unfortunately, data management systems, even when implemented properly, do not provide the basic flexibility or agility that end users often require.
Waiting for IT to get things implemented in a timely fashion is in a lot of cases not a realistic option. There’s also the issue of retrofitting all data sources into a data management system’s framework. This typically results in a lot of customisation and many one-off and unique processes which are costly to maintain and can prove a major headache during systems upgrades (think regression testing). All in all IT often has neither the time, appetite, or tools to optimally facilitate the business.
The result of this is that business teams will work around data management systems and revert to email/Excel solutions and simply work with the data they receive to get things done. This perpetuates the manual processing of data, introduces errors, lowers data quality and further complicates Excel solutions.
Security
Last but not least, data management processes that exist in this parallel world, outside of the enterprise systems, create real security issues (such as compliance issues, GDPR violations, contract breaches, etc.).
Data that is required to fulfill client obligations is often confidential but is sent across in unsecured emails. Emails end up in group mailboxes causing organisations to lose track of who has access to what.
Other solutions are to deliver data through portal and data sharing solutions. These offer secure transport mechanism but often credentials (username/passwords) are shared among colleagues and also through email. Apart from the fact that it introduces more manual work and consequently errors, it does not fundamentally improve the security situation.
What can you do?
There is a clear need for solutions that can bridge the gap between the manual and hard to automate data management processes on the one hand, and formal data management or operational systems on the other.
Ideally, organisations exchanging data would agree to execute on tight data delivery agreements and to implement highly automated exchange processes. Until we get there, a more pragmatic approach should probably be adopted, an approach where data as-is and a process of continuous improvement of data and relationship with supplier is accepted.
This means opening up processes and systems to deal with data in a structured manner. More concretely, this means organisations should follow clear workflow steps in collecting, validating, preparing and delivering data downstream. The end-result is flexible, agile and exception driven processes that provide visibility into each of the above steps and the ability to manually intervene only when required to do so.
In future blog posts we will describe how we look at each step of the above workflow, how this is operationalised and effectively supported by technology.
Mesoica’s data quality platform is specifically designed to help LPs and GPs manage their data efficiently. By using our platform, you can seamlessly collect, validate, and monitor data, enhancing communication and collaboration. Our scalable solution adapts to your organization's growing data needs, providing peace of mind and enabling you to become a truly data-driven organization. Start your journey today by visiting our website or contacting us to learn more about how Mesoica can empower your firm to continuously improve data quality.