Use Case
INREV SDDS Automation
A Use Case on Data Quality and Auditability with Mesoica
In the rapidly evolving landscape of real estate investment, handling data efficiently and accurately is key. Investment firms are often inundated with a variety of data formats, leading to labor-intensive and potentially error-prone manual processes. One such common data format is the INREV Standard Data Delivery Sheets (SDDS). We've worked with several investment firms that grappled with these challenges, seeking a more streamlined and reliable way to manage their data.
Our data quality platform is designed to automate and optimize data handling processes. In the following sections, we'll share how Mesoica can transform SDDS data management, enabling investment firms to unlock the full potential of real estate investment data, leading to improved risk management, asset allocation and financial outcomes.
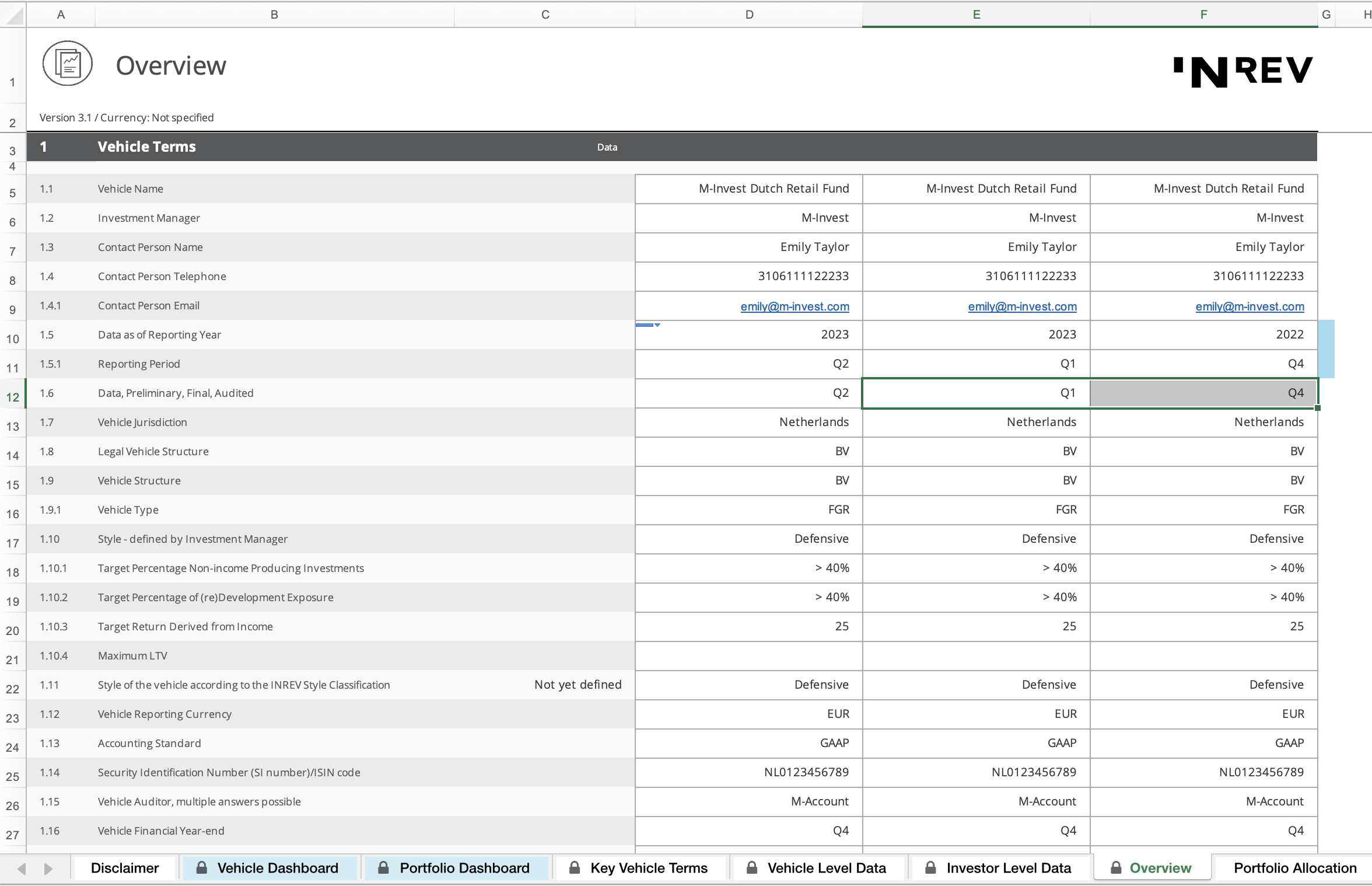
What is SDDS and why is it important?
SDDS is a standardization effort in the field of real estate investment (read more about it here: INREV SDDS Website). It ensures that key investment details are exchanged in a common format between fund managers and investors. In an ideal world this should lead to streamlined processes and operational efficiencies.
The SDDS provides crucial insights for investors, aiding in risk analysis, portfolio management, and financial planning. However, despite standardization, data still arrives in a format that's not immediately machine-friendly. It often requires manual intervention or Excel macros for transformation into a more suitable format for computer processing.
Moreover, the manual handling of data, especially when it involves receiving Excel files via emails, is not a robust or scalable data management solution. It's prone to errors, lacks traceability, and is difficult to maintain over time. Additionally, SDDSs are usually received quarterly, necessitating an efficient archival system for these data sets to facilitate historical analysis and ensure independent access to data.
We will discuss the challenges associated with handling SDDSs and how we tackled these in the following sections.
Problems with manual processes
We’ve often seen investment firms often grapple with the complexities of manual data extraction and data management from SDDS files. The issues inherent to these manual processes are numerous:
- Format Variations: Despite the standardization provided by SDDS, variations in format can still occur due to human error or slight differences in interpretation of the standard. This can lead to inconsistencies during data extraction and need to be harmonized later on.
- Error Proneness: Manual data extraction and processing are susceptible to errors. Mistakes can easily occur during data entry, extraction, transformation, and even during data storage. These errors can have far-reaching impacts, affecting the accuracy of analyses and decision-making processes.
- Lack of Workflow: Manual processes often lack a structured workflow. This absence of a workflow can lead to inefficient data handling, with potential for missed or duplicated tasks. It can also make it difficult to track the status of data processing tasks, resulting in administrative overload.
- Historical Data Maintenance: Manual systems frequently struggle with the maintenance of historical data. Without a robust system for archiving and retrieving past data, it becomes difficult to perform time-series analyses or trace back data for auditing or error correction.
These challenges underscore the importance of moving away from manual processes and adopting automated, standardized, and robust data management systems.
How we help
In response to these challenges, we introduced our Mesoica platform, a comprehensive solution that streamlines and automates data management processes. The Mesoica platform was designed to address the inherent issues associated with manual data extraction and processing, particularly in the context of SDDS data management.
To start, our solution automates the process of email ingestion, relieving the burden of manual data extraction. We designed a workflow where emails containing SDDS data are automatically ingested into our platform. This eliminates the need for manual downloading and handling of attachments, making the process significantly more efficient and less prone to human error. In this related article we explain how this is done https://mesoica.com/blog/from-manual-to-magical.
Additionally, we configured a series of data quality rules specifically tailored for raw SDDS data. These automated checks run on receipt of each individual SDDS spreadsheet and ensures that the incoming data adheres to established standards, significantly reducing the risk of data errors or inconsistencies.
Further enhancing the quality and reliability of the data, we deployed our in-flight data repair workflow. This involves an semi-automated process of identifying and correcting any issues with the data as it is being processed. This feature, paired with a 4-eyes sign off process, ensures that any data issues are swiftly and accurately addressed.
Finally, we streamlined the data transformation process. This entails converting the SDDS data into a format that can be easily ingested and utilized by the client's systems. Through our efficient transformation process, we ensure that the data is accurately converted and readily available for use.
In summary, we offer an automated, efficient, and reliable approach to managing SDDS data, significantly improving upon traditional, manual methods. Through automation, stringent data quality checks, and streamlined processes, we ensure that firms can effectively manage their SDDS data with confidence and ease.
Benefits of using a data quality and automation platform
The adoption of our Mesoica solution presented a shift for data management processes, delivering a significant benefits.
Firstly, automation was a key advantage. The Mesoica platform took over manual tasks such as email ingestion and data extraction, thereby reducing the time and effort required from the team. This enabled them to focus on more strategic tasks, elevating their productivity and effectiveness.
Secondly, the quality of data handling improved dramatically. Our automated checks, alerts, and reports ensured the accuracy and reliability of the SDDS data. Fewer errors and inconsistencies resulted in cleaner data, which led to more precise and dependable decision-making.
Thirdly, the Mesoica platform offered better control over the data management process. The in-flight data repair workflow coupled with the 4-eyes signoff process provided a systematic approach to identifying and resolving data issues. This proactive issue management ensured data integrity and minimized operational disruptions.
Furthermore, our solution enhanced the applicability of the SDDS data. The efficient transformation process allowed the SDDS data to be readily consumed by various systems, thereby widening its utility across the organization.
Finally, our solution significantly improved auditability. The automated processes, along with the Datasets Archive feature, ensured data traceability and allowed for historical analysis. This created a robust and auditable data management process, enhancing compliance and accountability.
In conclusion, the Mesoica solution not only addressed the challenges of managing SDDS data but also transformed the overall data management workflow. The benefits of automation, improved data quality, enhanced control, and auditability have set a new standard for efficient, reliable, and robust data management processes.
Mesoica’s data quality platform is specifically designed to help LPs and GPs manage their data efficiently. By using our platform, you can seamlessly collect, validate, and monitor data, enhancing communication and collaboration. Our scalable solution adapts to your organization's growing data needs, providing peace of mind and enabling you to become a truly data-driven organization. Start your journey today by visiting our website or contacting us to learn more about how Mesoica can empower your firm to continuously improve data quality.